Digit Detection with the Masterful CLI Trainer¶
In this guide, you will take a first look at Object Detection with the Masterful CLI Trainer to train a state of the art model.
This guide will use a simple dataset of handwritten digits called YYMNIST. This dataset was created by Yun Yang as the object detection equivalent of MNIST for classification. It’s a wonderful dataset for exploring object detection because its much faster to train and understand than the standard detection datasets like MSCOCO.
This guide will also demonstrate the power of using unlabeled data inside the Masterful CLI Trainer.
Define the Application¶
In this guide, your challenge is to build a CV model that can take an image with handwritten digits and localize the position of each digit and determine the class of each digit. This example is designed to get you familiar with using the Masterful CLI Trainer to perform different computer vision tasks.
Since you want to identify objects in the image, and their location, the CV task for this problem is object detection.
TL;DR¶
Don’t want to read the rest of this guide, and want to start training immediately? The following command shows you how to start training with Masterful, using a configuration file and dataset on S3.
masterful-train --config https://masterful-public.s3.us-west-1.amazonaws.com/datasets/yymnist/training_with_unlabeled.yaml
Prepare the Data¶
For this guide, you will use the YYMNIST dataset, which is an MNIST-like dataset for Object Detection. In this section, you will take the raw dataset and convert it into a format that Masterful can understand. Along the way, you will visualize a few examples of the data to see both the input to the model, as well as the predictions after training the model.
The original dataset can be generated by following the instructions here. This will generate a folder of images and a label.txt
file which contains the bounding box ground truth for each of the images. The label file has a simple format as follows:
# image_path x_min, y_min, x_max, y_max, class_id x_min, y_min ,..., class_id
This label format also happens to match the CSV format for Masterful Object Detection, which makes the data conversion task much easier. For your training and test sets, you can use the label file directly, and for your unlabeled set, you simply have to remove all of the bounding box and class information from each line.
Converting each dataset is typically a one-off operation that is different for every dataset you want to train with. For brevity, you can use the already converted dataset located at the public S3 bucket s3://masterful-public/datasets/yymnist/
. In this bucket, you will see the following files:
yymnist\
test.csv
train.csv
unlabeled.csv
training_with_unlabeled.yaml
training_no_unlabeled.yaml
images\
Explore the Data¶
You should always visually inspect your dataset to get a sense of what the model will see, and to roughly verify that your dataset conversion routine worked properly and you are not training with corrupted or incorrect data. This is especially important for object detection since it is very easy to generate incorrect bounding boxes during dataset conversion.
[6]:
# Install dependencies necessary to run the following
# code.
!pip install opencv-python-headless --quiet
!pip install masterful --quiet
# Import the packages used below.
import matplotlib.pyplot as plt
import os
import requests
import tarfile
import tempfile
import tensorflow as tf
import urllib.request
# Import and activate the Masterful package.
import masterful
masterful = masterful.activate()
# Helper function to visualize bounding boxes from the dataset
# on the source image.
from masterful.data.visualize import create_annotated_image
# Helper function to display a progress when downloading
# a file using HTTP.
from masterful.utils.downloader import progress_bar_factory
# This is necessary for running inside of Colab/Jupyter,
# since the CLI trainer is run outside of the kernel
# (as a script command).
gpus = tf.config.experimental.list_physical_devices('GPU')
for gpu in gpus:
tf.config.experimental.set_memory_growth(gpu, True)
MASTERFUL: Your account has been successfully activated. Masterful v0.5.2 is loaded.
[7]:
DATASET_ROOT = "https://masterful-public.s3.us-west-1.amazonaws.com/datasets/yymnist/"
TRAINING_CSV_URL = os.path.join(DATASET_ROOT, "train.csv")
with tempfile.TemporaryDirectory() as temp_directory:
training_csv_name = os.path.join(temp_directory, "train.csv")
_ = urllib.request.urlretrieve(TRAINING_CSV_URL, training_csv_name)
examples = []
with open(training_csv_name) as training_csv:
examples = training_csv.readlines()
f, axarr = plt.subplots(2, 2, figsize=(15,15))
_ = axarr[0, 0].imshow(create_annotated_image(examples[0], DATASET_ROOT))
_ = axarr[0, 1].imshow(create_annotated_image(examples[1], DATASET_ROOT))
_ = axarr[1, 0].imshow(create_annotated_image(examples[2], DATASET_ROOT))
_ = axarr[1, 1].imshow(create_annotated_image(examples[3], DATASET_ROOT))
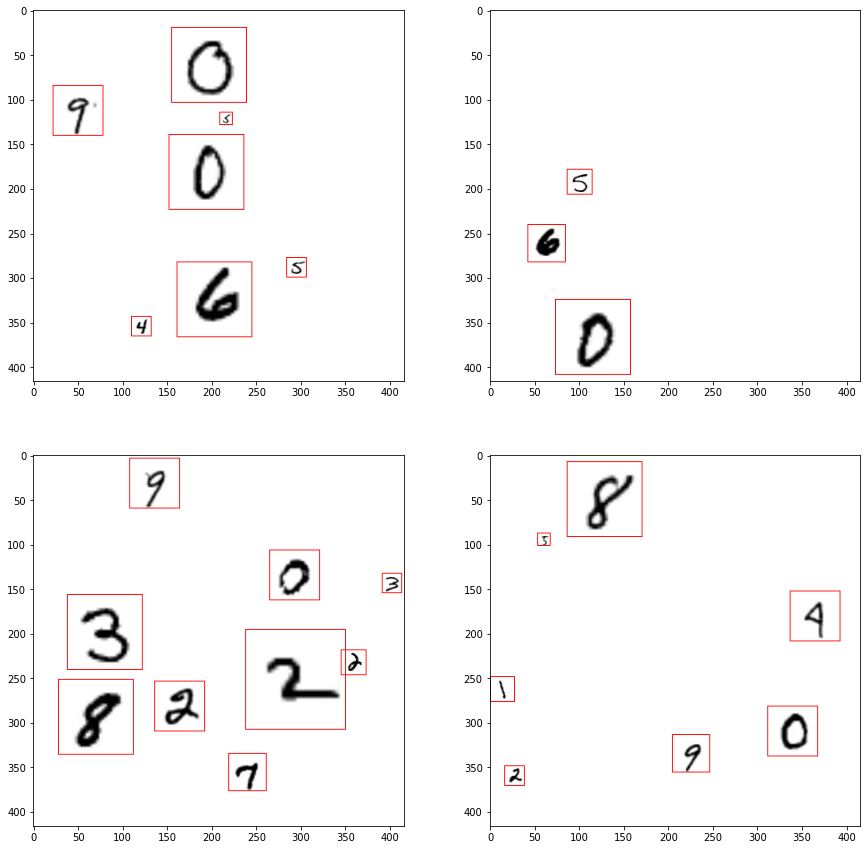
Configure the CLI Trainer¶
The Masterful CLI Trainer is a command line tool that trains a production quality model with no code required. The Masterful CLI Trainer take a YAML configuration file as input. The configuration file fully specifies everything necessary for training, including the dataset, model, export formats, and evaluation metrics.
Choosing a Model¶
Masterful provides many different state of the art object detection models that you can choose from. In general, choosing a model can have different constraints than training the model (are you deploying on server or edge? Runtime or latency constraints? Memory constraints?). You generally want to choose a model that is large enough to fit your data, but not so large as to overfit the training data and “memorize” the results, which can lead to poor generalization performance.
The Masterful Object Detection Model Zoo supports the following model architectures.
Model Name |
Year |
Description |
---|---|---|
|
2018 |
Single-Shot Detector with MobileNet V1 Feature Pyramid backbone, from the paper Focal Loss for Dense Object Detection |
|
2018 |
Single-Shot Detector with MobileNet V2 backbone, from the paper Focal Loss for Dense Object Detection |
|
2018 |
Single-Shot Detector with MobileNet V2 Feature Pyramid Lite backbone, from the paper Focal Loss for Dense Object Detection |
|
2018 |
Single-Shot Detector with ResNet-50 V1 Feature Pyramid backbone, from the paper Focal Loss for Dense Object Detection |
|
2018 |
Single-Shot Detector with ResNet-101 V1 Feature Pyramid backbone, from the paper Focal Loss for Dense Object Detection |
|
2018 |
Single-Shot Detector with ResNet-152 V1 Feature Pyramid backbone, from the paper Focal Loss for Dense Object Detection |
|
2018 |
RetinaNet with ResNet-50 V1 Feature Pyramid backbone, from the paper Focal Loss for Dense Object Detection |
|
2018 |
RetinaNet with ResNet-101 V1 Feature Pyramid backbone, from the paper Focal Loss for Dense Object Detection |
|
2018 |
RetinaNet with ResNet-152 V1 Feature Pyramid backbone, from the paper Focal Loss for Dense Object Detection |
|
2019 |
EfficientDet-D0 model from the paper EfficientDet: Scalable and Efficient Object Detection |
|
2019 |
EfficientDet-D1 model from the paper EfficientDet: Scalable and Efficient Object Detection |
|
2019 |
EfficientDet-D2 model from the paper EfficientDet: Scalable and Efficient Object Detection |
|
2019 |
EfficientDet-D3 model from the paper EfficientDet: Scalable and Efficient Object Detection |
|
2019 |
EfficientDet-D4 model from the paper EfficientDet: Scalable and Efficient Object Detection |
|
2019 |
EfficientDet-D5 model from the paper EfficientDet: Scalable and Efficient Object Detection |
|
2019 |
EfficientDet-D6 model from the paper EfficientDet: Scalable and Efficient Object Detection |
|
2019 |
EfficientDet-D7 model from the paper EfficientDet: Scalable and Efficient Object Detection |
|
2016 |
Faster R-CNN model with a ResNet-50 V1 backbone, from the paper Faster R-CNN: Towards Real-Time Object Detection with Region Proposal Networks |
|
2016 |
Faster R-CNN model with a ResNet-101 V1 backbone, from the paper Faster R-CNN: Towards Real-Time Object Detection with Region Proposal Networks |
|
2016 |
Faster R-CNN model with a ResNet-152 V1 backbone, from the paper Faster R-CNN: Towards Real-Time Object Detection with Region Proposal Networks |
|
2016 |
Faster R-CNN model with an Inception ResNet V2 backbone, from the paper Faster R-CNN: Towards Real-Time Object Detection with Region Proposal Networks |
|
2019 |
CenterNet model with an Hourglass-104 backbone, from the paper Objects as Points |
|
2019 |
CenterNet model with a ResNet-50 V1 backbone, from the paper Objects as Points |
|
2019 |
CenterNet model with a ResNet-101 V1 backbone, from the paper Objects as Points |
|
2019 |
CenterNet model with a ResNet-50 V2 backbone, from the paper Objects as Points |
|
2019 |
CenterNet model with a MobileNet V2 backbone, from the paper Objects as Points |
In the example below, you will select a SSD model with a MobileNet-V2 backbone. This model is great for small datasets or for deploying to edge devices, as it is tuned for quick inference at a small cost to overall accuracy.
The Configuration File¶
The following section is a condensed YAML configuration file for training against this dataset with NO unlabeled data. It points to the dataset created above, and selects a ssd_mobilenet_v2
model, which is a good model for small datasets and edge inference use cases.
Note all of the model artifacts will be saved to the directory ~/model_output
, which you can configure in the output
section below.
The original YAML file can be found here.
dataset:
root_path: https://masterful-public.s3.us-west-1.amazonaws.com/datasets/yymnist
splits: [train, test]
label_format: detection_csv
optimize: True
model:
architecture: ssd_mobilenet_v2
num_classes: 10
input_shape: [416,416,3]
training:
task: detection
training_split: train
output:
formats: [saved_model, onnx]
path: ~/model_output
evaluation:
split: test
Train the Model¶
Training the model involves simply running the Masterful CLI Trainer (masterful-train
) against the YAML file on S3. As a first step, you will train the model without any additional unlabeled data. This way, you can see the performance improvement later on when you add unlabeled data.
Below, you will setup the CLI and configuration file to run in this notebook. However, if you want to train this model outside of the notebook, against the dataset on S3, you can run:
masterful-train --config https://masterful-public.s3.us-west-1.amazonaws.com/datasets/yymnist/training_no_unlabeled.yaml
NOTE: Object detection models are quite large. Depending on your GPU, this could take several hours to complete, so plan accordingly.
[1]:
# Use the Masterful CLI to train the model without unlabeled data
!masterful-train --config https://masterful-public.s3.us-west-1.amazonaws.com/datasets/yymnist/training_no_unlabeled.yaml
MASTERFUL: Your account has been successfully activated. Masterful v0.5.2 is loaded.
MASTERFUL [12:17:35]: Training with configuration 'https://masterful-public.s3.us-west-1.amazonaws.com/datasets/yymnist/training_no_unlabeled.yaml':
---------- ----------------------------------------------------------------------------------
dataset root_path https://masterful-public.s3.us-west-1.amazonaws.com/datasets/yymnist
splits ['train', 'test']
label_format detection_csv
optimize True
model architecture ssd_mobilenet_v2
num_classes 10
input_shape [416, 416, 3]
training task detection
training_split train
output formats ['saved_model', 'onnx']
path ~/model_output
evaluation split test
---------- ----------------------------------------------------------------------------------
MASTERFUL [12:17:36]: Building model 'ssd_mobilenet_v2'...
Downloading Weights:100% (46042990 of 46042990) || Elapsed Time: 0:00:05 Time: 0:00:05
MASTERFUL [12:17:47]: Using model ssd_mobilenet_v2 with:
MASTERFUL [12:17:47]: 4795759 total parameters
MASTERFUL [12:17:47]: 4758191 trainable parameters
MASTERFUL [12:17:47]: 37568 untrainable parameters
MASTERFUL [12:17:49]: Dataset Summary:
MASTERFUL [12:17:49]: Training Dataset: 900 examples.
MASTERFUL [12:17:49]: Validation Dataset: 0 examples.
MASTERFUL [12:17:49]: Test Dataset: 100 examples.
MASTERFUL [12:17:49]: Unlabeled Dataset: 0 examples.
MASTERFUL [12:17:49]: Training Dataset Analysis:
100%|█████████████████████████████████████████| 900/900 [00:16<00:00, 53.40it/s]
MASTERFUL [12:18:06]: Training dataset analysis finished at 12:18:06 in 17 seconds (17s), returned:
----------------------------- ----------------------------------------
Total Examples 900
Label Counts 0 494
1 616
2 552
3 533
4 522
5 440
6 581
7 555
8 491
9 549
Label Distribution 0 0.0926308
1 0.115507
2 0.103506
3 0.0999437
4 0.0978811
5 0.0825052
6 0.108944
7 0.104069
8 0.0920683
9 0.102944
Balanced Yes
Per Channel Mean [251.53575897 251.53575897 251.53575897]
Per Channel StdDev [26.2797294 26.2797294 26.2797294]
Min Height 416
Min Width 416
Max Height 416
Max Width 416
Average Height 416
Average Width 416
Largest Image (416, 416, 3)
Smallest Image (416, 416, 3)
Duplicates 0
Average Object Area 3565
Smallest Object Area 195
Largest Object Area 12544
Num Small Objects 2216
Num Medium Objects 2415
Num Large Objects 702
Num Empty Images (No Labels): 0
Total Objects 5333
----------------------------- ----------------------------------------
MASTERFUL [12:18:06]: Test Dataset Analysis:
100%|█████████████████████████████████████████| 100/100 [00:01<00:00, 50.28it/s]
MASTERFUL [12:18:08]: Test dataset analysis finished at 12:18:08 in 2 seconds (2s), returned:
----------------------------- ----------------------------------------
Total Examples 100
Label Counts 0 70
1 57
2 44
3 62
4 74
5 60
6 66
7 78
8 55
9 59
Label Distribution 0 0.112
1 0.0912
2 0.0704
3 0.0992
4 0.1184
5 0.096
6 0.1056
7 0.1248
8 0.088
9 0.0944
Balanced Yes
Per Channel Mean [251.62994095 251.62994095 251.62994095]
Per Channel StdDev [26.10288871 26.10288871 26.10288871]
Min Height 416
Min Width 416
Max Height 416
Max Width 416
Average Height 416
Average Width 416
Largest Image (416, 416, 3)
Smallest Image (416, 416, 3)
Duplicates 0
Average Object Area 3259
Smallest Object Area 195
Largest Object Area 12544
Num Small Objects 270
Num Medium Objects 290
Num Large Objects 65
Num Empty Images (No Labels): 0
Total Objects 625
----------------------------- ----------------------------------------
MASTERFUL [12:18:08]: Cross-Dataset Analysis:
MASTERFUL [12:18:08]: Cross-Dataset analysis finished at 12:18:08 in 0 seconds (0s), returned:
----- --------
train train 0
test 0
test train 0
test 0
----- --------
MASTERFUL [12:18:08]: Meta-Learning architecture parameters...
MASTERFUL [12:18:08]: Architecture learner finished at 12:18:08 in 0 seconds (0s), returned:
------------------------------ -----------------------------------------------------
task Task.DETECTION
num_classes 10
ensemble_multiplier 1
custom_objects
model_config ssd {
num_classes: 10
image_resizer {
fixed_shape_resizer {
height: 416
width: 416
}
}
feature_extractor {
type: "ssd_mobilenet_v2_keras"
depth_multiplier: 1.0
min_depth: 16
conv_hyperparams {
regularizer {
l2_regularizer {
weight: 3.9999998989515007e-05
}
}
initializer {
truncated_normal_initializer {
mean: 0.0
stddev: 0.029999999329447746
}
}
activation: RELU_6
batch_norm {
decay: 0.9700000286102295
center: true
scale: true
epsilon: 0.0010000000474974513
train: true
}
}
override_base_feature_extractor_hyperparams: true
}
box_coder {
faster_rcnn_box_coder {
y_scale: 10.0
x_scale: 10.0
height_scale: 5.0
width_scale: 5.0
}
}
matcher {
argmax_matcher {
matched_threshold: 0.5
unmatched_threshold: 0.5
ignore_thresholds: false
negatives_lower_than_unmatched: true
force_match_for_each_row: true
use_matmul_gather: true
}
}
similarity_calculator {
iou_similarity {
}
}
box_predictor {
convolutional_box_predictor {
conv_hyperparams {
regularizer {
l2_regularizer {
weight: 3.9999998989515007e-05
}
}
initializer {
random_normal_initializer {
mean: 0.0
stddev: 0.009999999776482582
}
}
activation: RELU_6
batch_norm {
decay: 0.9700000286102295
center: true
scale: true
epsilon: 0.0010000000474974513
train: true
}
}
min_depth: 0
max_depth: 0
num_layers_before_predictor: 0
use_dropout: false
dropout_keep_probability: 0.800000011920929
kernel_size: 1
box_code_size: 4
apply_sigmoid_to_scores: false
class_prediction_bias_init: -4.599999904632568
}
}
anchor_generator {
ssd_anchor_generator {
num_layers: 6
min_scale: 0.20000000298023224
max_scale: 0.949999988079071
aspect_ratios: 1.0
aspect_ratios: 2.0
aspect_ratios: 0.5
aspect_ratios: 3.0
aspect_ratios: 0.33329999446868896
}
}
post_processing {
batch_non_max_suppression {
score_threshold: 9.99999993922529e-09
iou_threshold: 0.6000000238418579
max_detections_per_class: 100
max_total_detections: 100
}
score_converter: SIGMOID
}
normalize_loss_by_num_matches: true
loss {
localization_loss {
weighted_smooth_l1 {
delta: 1.0
}
}
classification_loss {
weighted_sigmoid_focal {
gamma: 2.0
alpha: 0.75
}
}
classification_weight: 1.0
localization_weight: 1.0
}
encode_background_as_zeros: true
normalize_loc_loss_by_codesize: true
inplace_batchnorm_update: true
freeze_batchnorm: false
}
backbone_only False
input_shape (416, 416, 3)
input_range ImageRange.ZERO_255
input_dtype <dtype: 'float32'>
input_channels_last True
prediction_logits True
prediction_dtype <dtype: 'float32'>
prediction_structure TensorStructure.DICT
prediction_shape
total_parameters
total_trainable_parameters
total_non_trainable_parameters
------------------------------ -----------------------------------------------------
MASTERFUL [12:18:08]: Meta-learning training dataset parameters...
MASTERFUL [12:18:09]: Training dataset learner finished at 12:18:09 in 1 seconds (1s), returned:
------------------------- -----------------------------
num_classes 10
task Task.DETECTION
image_shape (416, 416, 3)
image_range ImageRange.ZERO_255
image_dtype <dtype: 'float32'>
image_channels_last True
label_dtype <dtype: 'float32'>
label_shape (12, 15)
label_structure TensorStructure.SINGLE_TENSOR
label_sparse False
label_bounding_box_format BoundingBoxFormat.TENSORFLOW
------------------------- -----------------------------
MASTERFUL [12:18:09]: Meta-learning test dataset parameters...
MASTERFUL [12:18:10]: Test dataset learner finished at 12:18:10 in 1 seconds (1s), returned:
------------------------- -----------------------------
num_classes 10
task Task.DETECTION
image_shape (416, 416, 3)
image_range ImageRange.ZERO_255
image_dtype <dtype: 'float32'>
image_channels_last True
label_dtype <dtype: 'float32'>
label_shape (11, 15)
label_structure TensorStructure.SINGLE_TENSOR
label_sparse False
label_bounding_box_format BoundingBoxFormat.TENSORFLOW
------------------------- -----------------------------
MASTERFUL [12:18:10]: Meta-Learning optimization parameters...
Callbacks: 100%|███████████████████████████████| 5/5 [01:23<00:00, 16.63s/steps]
MASTERFUL [12:19:33]: Optimization learner finished at 12:19:33 in 84 seconds (1m 24s), returned:
----------------------- -----------------------------------------------------------------
batch_size 16
drop_remainder True
epochs 1000000
learning_rate 0.0008838834473863244
learning_rate_schedule
learning_rate_callback <keras.callbacks.ReduceLROnPlateau object at 0x7f2695fe89e8>
warmup_learning_rate 1e-06
warmup_epochs 5
optimizer <tensorflow_addons.optimizers.lamb.LAMB object at 0x7f2695fe8208>
loss
loss_weights
early_stopping_callback <keras.callbacks.EarlyStopping object at 0x7f2695eec630>
metrics
readonly_callbacks
----------------------- -----------------------------------------------------------------
MASTERFUL [12:19:36]: Meta-Learning Regularization Parameters...
MASTERFUL [12:19:50]: Warming up model for analysis.
MASTERFUL [12:19:50]: Warming up batch norm statistics (this could take a few minutes).
MASTERFUL [12:19:53]: Warming up training for 250 steps.
100%|██████████████████████████████████████| 250/250 [01:58<00:00, 2.10steps/s]
MASTERFUL [12:21:52]: Validating batch norm statistics after warmup for stability (this could take a few minutes).
MASTERFUL [12:21:54]: Analyzing baseline model performance. Training until validation loss stabilizes...
Baseline Training: 100%|█████████████████| 2530/2530 [10:29<00:00, 4.02steps/s]
MASTERFUL [12:32:46]: Baseline training complete.
MASTERFUL [12:32:46]: Meta-Learning Basic Data Augmentations...
Node 1/4: 100%|██████████████████████████| 1100/1100 [04:31<00:00, 4.05steps/s]
Node 2/4: 100%|██████████████████████████| 1100/1100 [04:31<00:00, 4.06steps/s]
Node 3/4: 100%|██████████████████████████| 1100/1100 [04:24<00:00, 4.15steps/s]
Node 4/4: 100%|██████████████████████████| 1100/1100 [04:25<00:00, 4.15steps/s]
MASTERFUL [12:51:45]: Meta-Learning Data Augmentation Clusters...
Distance Analysis: 100%|███████████████████| 143/143 [02:16<00:00, 1.05steps/s]
Node 1/10: 100%|█████████████████████████| 1100/1100 [05:55<00:00, 3.10steps/s]
Node 2/10: 100%|█████████████████████████| 1100/1100 [05:56<00:00, 3.09steps/s]
Node 3/10: 100%|█████████████████████████| 1100/1100 [05:55<00:00, 3.10steps/s]
Node 4/10: 100%|█████████████████████████| 1100/1100 [05:57<00:00, 3.08steps/s]
Node 5/10: 100%|█████████████████████████| 1100/1100 [05:56<00:00, 3.08steps/s]
Distance Analysis: 100%|█████████████████████| 66/66 [01:08<00:00, 1.04s/steps]
Node 6/10: 100%|█████████████████████████| 1100/1100 [06:18<00:00, 2.91steps/s]
Node 7/10: 100%|█████████████████████████| 1100/1100 [06:18<00:00, 2.91steps/s]
Node 8/10: 100%|█████████████████████████| 1100/1100 [06:17<00:00, 2.91steps/s]
Node 9/10: 100%|█████████████████████████| 1100/1100 [06:19<00:00, 2.90steps/s]
Node 10/10: 100%|████████████████████████| 1100/1100 [06:18<00:00, 2.90steps/s]
MASTERFUL [14:00:04]: Meta-Learning Label Based Regularization...
Node 1/2: 100%|██████████████████████████| 1100/1100 [05:58<00:00, 3.07steps/s]
Node 2/2: 100%|██████████████████████████| 1100/1100 [06:01<00:00, 3.04steps/s]
MASTERFUL [14:12:50]: Meta-Learning Weight Based Regularization...
MASTERFUL [14:12:51]: Analysis finished in 113.08219845692317 minutes.
MASTERFUL [14:12:51]: Learned parameters bandana-vigorous-daffodil saved at /home/sam/.masterful/policies/bandana-vigorous-daffodil.
MASTERFUL [14:12:51]: Regularization learner finished at 14:12:51 in 6798 seconds (1h 53m 18s), returned:
------------------------- -----------------------------------------------
shuffle_buffer_size 810
mirror 0.0
rot90 0.0
rotate 0
mixup 0.75
cutmix 0.5
label_smoothing 0
hsv_cluster 4
hsv_cluster_to_index [[11 11 11 11 11 11]
[11 11 11 11 11 11]
[11 11 11 11 11 11]
[11 11 11 11 11 11]
[11 11 11 11 11 11]]
hsv_magnitude_table [[ 0 10 20 30 40 50 60 70 80 90 100]
[ 0 10 20 30 40 50 60 70 80 90 100]
[ 0 10 20 30 40 50 60 70 80 90 100]
[ 0 10 20 30 40 50 60 70 80 90 100]
[ 0 10 20 30 40 50 60 70 80 90 100]]
contrast_cluster 4
contrast_cluster_to_index [[11 11 11 11 11 11]
[11 11 11 11 11 11]
[11 11 11 11 11 11]
[11 11 11 11 11 11]
[11 11 11 11 11 11]
[11 11 11 11 11 11]]
contrast_magnitude_table [[ 0 10 20 30 40 50 60 70 80 90 100]
[ 0 10 20 30 40 50 60 70 80 90 100]
[ 0 10 20 30 40 50 60 70 80 90 100]
[ 0 10 20 30 40 50 60 70 80 90 100]
[ 0 10 20 30 40 50 60 70 80 90 100]
[ 0 10 20 30 40 50 60 70 80 90 100]]
blur_cluster 4
blur_cluster_to_index [[11 11 11 11 11 11]
[11 11 11 11 11 11]]
blur_magnitude_table [[ 0 10 20 30 40 50 60 70 80 90 100]
[ 0 10 20 30 40 50 60 70 80 90 100]]
spatial_cluster 0
spatial_cluster_to_index [[11 11 11 11 11 11]
[11 11 11 11 11 11]
[11 11 11 11 11 11]
[11 11 11 11 11 11]
[11 11 11 11 11 11]
[11 11 11 11 11 11]]
spatial_magnitude_table [[ 0 10 20 30 40 50 60 70 80 90 100]
[ 0 10 20 30 40 50 60 70 80 90 100]
[ 0 10 20 30 40 50 60 70 80 90 100]
[ 0 10 20 30 40 50 60 70 80 90 100]
[ 0 10 20 30 40 50 60 70 80 90 100]
[ 0 10 20 30 40 50 60 70 80 90 100]]
synthetic_proportion [0.0]
------------------------- -----------------------------------------------
MASTERFUL [14:12:51]: Learning SSL parameters...
MASTERFUL [14:12:52]: SSL learner finished at 14:12:52 in 1 seconds (1s), returned:
---------- --
algorithms []
---------- --
MASTERFUL [14:12:52]: Training model with semi-supervised learning disabled.
MASTERFUL [14:12:53]: Performing basic dataset analysis.
MASTERFUL [14:13:03]: Masterful will use 90 labeled examples as a validation set since no validation data was provided.
MASTERFUL [14:13:03]: Training model with:
MASTERFUL [14:13:03]: 810 labeled examples.
MASTERFUL [14:13:03]: 90 validation examples.
MASTERFUL [14:13:03]: 0 synthetic examples.
MASTERFUL [14:13:03]: 0 unlabeled examples.
MASTERFUL [14:13:04]: Training model with learned parameters bandana-vigorous-daffodil in two phases.
MASTERFUL [14:13:04]: The first phase is supervised training with the learned parameters.
MASTERFUL [14:13:04]: The second phase is semi-supervised training to boost performance.
MASTERFUL [14:13:08]: Warming up model for supervised training.
MASTERFUL [14:13:09]: Warming up batch norm statistics (this could take a few minutes).
MASTERFUL [14:13:12]: Warming up training for 250 steps.
100%|██████████████████████████████████████| 250/250 [01:59<00:00, 2.09steps/s]
MASTERFUL [14:15:11]: Validating batch norm statistics after warmup for stability (this could take a few minutes).
MASTERFUL [14:15:13]: Starting Phase 1: Supervised training until the validation loss stabilizes...
Supervised Training: 100%|███████████████| 2805/2805 [14:25<00:00, 3.24steps/s]
MASTERFUL [14:30:01]: Semi-Supervised training disabled in parameters.
MASTERFUL [14:30:03]: Training complete in 16.970766989390054 minutes.
MASTERFUL [14:30:37]: Saving model output to /home/sam/model_output/session-00341.
MASTERFUL [14:30:48]: Saving saved_model output to /home/sam/model_output/session-00341/saved_model
MASTERFUL [14:31:26]: Saving onnx output to /home/sam/model_output/session-00341/onnx
MASTERFUL [14:32:32]: Saving regularization params to /home/sam/model_output/session-00341/regularization.params.
MASTERFUL [14:32:32]: ************************************
MASTERFUL [14:32:32]: Evaluating model on 100 examples from the 'test' dataset split:
Evaluating: 100%|█████████████████████████████| 100/100 [00:04<00:00, 24.47it/s]
MASTERFUL [14:32:36]: Loss: 0.2189
MASTERFUL [14:32:36]: Loss/Localization Loss: 0.0251
MASTERFUL [14:32:36]: Loss/Classification Loss: 0.1134
MASTERFUL [14:32:36]: Loss/Regularization Loss: 0.0803
MASTERFUL [14:32:36]: Loss/Total Loss: 0.2189
MASTERFUL [14:32:36]: Calculating MSCOCO evaluation metrics:
MSCOCO Metrics: 100%|█████████████████████████| 100/100 [00:13<00:00, 7.61it/s]
MASTERFUL [14:32:49]: DetectionBoxes_Precision/mAP: 0.7454
MASTERFUL [14:32:49]: DetectionBoxes_Precision/mAP@.50IOU: 0.9290
MASTERFUL [14:32:49]: DetectionBoxes_Precision/mAP@.75IOU: 0.8823
MASTERFUL [14:32:49]: DetectionBoxes_Precision/mAP (small): 0.6022
MASTERFUL [14:32:49]: DetectionBoxes_Precision/mAP (medium): 0.8318
MASTERFUL [14:32:49]: DetectionBoxes_Precision/mAP (large): 0.9343
MASTERFUL [14:32:49]: DetectionBoxes_Recall/AR@1: 0.6101
MASTERFUL [14:32:49]: DetectionBoxes_Recall/AR@10: 0.7949
MASTERFUL [14:32:49]: DetectionBoxes_Recall/AR@100: 0.7949
MASTERFUL [14:32:49]: DetectionBoxes_Recall/AR@100 (small): 0.6781
MASTERFUL [14:32:49]: DetectionBoxes_Recall/AR@100 (medium): 0.8705
MASTERFUL [14:32:49]: DetectionBoxes_Recall/AR@100 (large): 0.9479
MASTERFUL [14:32:49]: DetectionBoxes_PerformanceByCategory/mAP/0: 0.7744
MASTERFUL [14:32:49]: DetectionBoxes_PerformanceByCategory/mAP/1: 0.7481
MASTERFUL [14:32:49]: DetectionBoxes_PerformanceByCategory/mAP/2: 0.7269
MASTERFUL [14:32:49]: DetectionBoxes_PerformanceByCategory/mAP/3: 0.7851
MASTERFUL [14:32:49]: DetectionBoxes_PerformanceByCategory/mAP/4: 0.7444
MASTERFUL [14:32:49]: DetectionBoxes_PerformanceByCategory/mAP/5: 0.7149
MASTERFUL [14:32:49]: DetectionBoxes_PerformanceByCategory/mAP/6: 0.7831
MASTERFUL [14:32:49]: DetectionBoxes_PerformanceByCategory/mAP/7: 0.7767
MASTERFUL [14:32:49]: DetectionBoxes_PerformanceByCategory/mAP/8: 0.6802
MASTERFUL [14:32:49]: DetectionBoxes_PerformanceByCategory/mAP/9: 0.7204
Confusion Matrix: 100%|███████████████████████| 100/100 [00:10<00:00, 9.17it/s]
MASTERFUL [14:33:00]: Confusion Matrix:
MASTERFUL [14:33:00]: | 0| 1| 2| 3| 4| 5| 6| 7| 8| 9| background|
MASTERFUL [14:33:00]: 0| 64| 0| 0| 0| 0| 0| 0| 0| 1| 0| 5|
MASTERFUL [14:33:00]: 1| 0| 55| 0| 0| 0| 0| 0| 0| 0| 0| 2|
MASTERFUL [14:33:00]: 2| 0| 0| 34| 5| 0| 0| 0| 0| 0| 1| 4|
MASTERFUL [14:33:00]: 3| 0| 0| 2| 57| 0| 0| 0| 0| 0| 1| 2|
MASTERFUL [14:33:00]: 4| 0| 1| 0| 0| 59| 0| 1| 2| 0| 5| 6|
MASTERFUL [14:33:00]: 5| 0| 0| 1| 0| 1| 53| 0| 0| 1| 0| 4|
MASTERFUL [14:33:00]: 6| 0| 1| 0| 0| 0| 0| 62| 0| 1| 0| 2|
MASTERFUL [14:33:00]: 7| 0| 1| 2| 1| 1| 0| 0| 63| 0| 6| 4|
MASTERFUL [14:33:00]: 8| 1| 0| 0| 2| 0| 2| 2| 0| 40| 3| 5|
MASTERFUL [14:33:00]: 9| 0| 0| 0| 1| 2| 0| 0| 1| 0| 49| 6|
MASTERFUL [14:33:00]: background| 0| 2| 2| 4| 3| 2| 0| 4| 2| 4| 0|
MASTERFUL [14:33:00]: Confusion matrix columns represent the prediction labels and the rows represent the real labels.
MASTERFUL [14:33:00]: Saving evaluation metrics to /home/sam/model_output/session-00341/evaluation_metrics.csv.
MASTERFUL [14:33:00]: Saving confusion matrix to /home/sam/model_output/session-00341/confusion_matrix.csv.
MASTERFUL [14:33:00]: Total elapsed training time: 135 minutes (2h 15m 25s).
MASTERFUL [14:33:00]: Launch masterful-gui to visualize the training results: policy name 'bandana-vigorous-daffodil'
Analyze the Results¶
At the end of training, Masterful will evaluate your model based on the test (sometimes called holdout) dataset you specified in the evaluation
section of your configuration file. The evaluation results are different for each task, since every computer vision task has both general metrics (such as loss) as well as task-specific metrics (IoU for semantic segmentation for example). These results are printed to the console, as well as saved into a CSV file in the output directory specified in
your configuration file.
Object Detection Evaluation Metrics¶
For Object Detection, Masterful reports three main categories of metrics: Model Loss, COCO Metrics, and Confusion matrix.
Model Loss¶
The first set of metrics Masterful reports is the overall loss of the model. For object detection models, Masterful reports the total loss as well as any sub-losses that are specific to the model architecture. For example, the loss is typically composed of a localization loss (which reflects the location and size of the bounding box) and a classification loss (which reflects which object is in the box). Different models have different sub-losses, and you can gauge how your model is doing on the different tasks based on the final results of these sub-metrics.
The following is the loss results from the above training run (this might be different if you rerun the above cells):
************************************
MASTERFUL [14:32:32]: Evaluating model on 100 examples from the 'test' dataset split:
Evaluating: 100%|█████████████████████████████| 100/100 [00:04<00:00, 24.47it/s]
MASTERFUL [14:32:36]: Loss: 0.2189
MASTERFUL [14:32:36]: Loss/Localization Loss: 0.0251
MASTERFUL [14:32:36]: Loss/Classification Loss: 0.1134
MASTERFUL [14:32:36]: Loss/Regularization Loss: 0.0803
MASTERFUL [14:32:36]: Loss/Total Loss: 0.2189
As you can see in the above output, the total loss of the model is MASTERFUL [14:32:36]: Loss: 0.2189
(which matches the Loss/Total Loss
output as well). You can check the localization and classification loss as well, and you can see that the classification loss 0.1134
is slightly worse than the localization loss 0.0251
. These seem like pretty good loss values. They are relatively low and close to zero, which is the goal of the optimizer in machine learning training. However,
it’s really difficult to understand intuitively what these mean in terms of your models overall performance. Are these good values? Or bad ones? Let’s dive into the rest of the metrics to answer these questions.
COCO Metrics¶
Common Objects in Context (COCO) is a large-scale object detection, segmentation, and captioning dataset. COCO has several features:
Object segmentation
Recognition in context
Superpixel stuff segmentation
330K images (>200K labeled)
1.5 million object instances
80 object categories
91 stuff categories
5 captions per image
250,000 people with keypoints
The dataset itself is used in many research papers as a standard object detection benchmark. Importantly, COCO also defines a standard set of metrics for evaluating object detection models, commonly called the COCO (MS-COCO) Evaluation Metrics.
The following 12 metrics are used for characterizing the performance of an object detector as part of the COCO detection challenge:
Unless otherwise specified, AP and AR are averaged over multiple Intersection over Union (IoU) values. Specifically we use 10 IoU thresholds of .50:.05:.95. This is a break from tradition, where AP is computed at a single IoU of .50 (which corresponds to our metric APIoU=.50). Averaging over IoUs rewards detectors with better localization.
AP is averaged over all categories. Traditionally, this is called “mean average precision” (mAP). We make no distinction between AP and mAP (and likewise AR and mAR) and assume the difference is clear from context.
AP (averaged across all 10 IoU thresholds and all 80 categories) will determine the challenge winner. This should be considered the single most important metric when considering performance on COCO. In COCO, there are more small objects than large objects. Specifically: approximately 41% of objects are small (area < 322), 34% are medium (322 < area < 962), and 24% are large (area > 962). Area is measured as the number of pixels in the segmentation mask.
AR is the maximum recall given a fixed number of detections per image, averaged over categories and IoUs. AR is related to the metric of the same name used in proposal evaluation but is computed on a per-category basis.
All metrics are computed allowing for at most 100 top-scoring detections per image (across all categories).
The evaluation metrics for detection with bounding boxes and segmentation masks are identical in all respects except for the IoU computation (which is performed over boxes or masks, respectively).
For more information about things like Average Precision and Recall and IoU in the context of Object Detection, there are several good walkthroughs below with worked examples. They will give you a better intuitive sense of what the metrics are trying to convey:
Below, you will find the COCO metrics for the model you trained above:
MASTERFUL [14:32:36]: Calculating MSCOCO evaluation metrics:
MSCOCO Metrics: 100%|█████████████████████████| 100/100 [00:13<00:00, 7.61it/s]
MASTERFUL [14:32:49]: DetectionBoxes_Precision/mAP: 0.7454
MASTERFUL [14:32:49]: DetectionBoxes_Precision/mAP@.50IOU: 0.9290
MASTERFUL [14:32:49]: DetectionBoxes_Precision/mAP@.75IOU: 0.8823
MASTERFUL [14:32:49]: DetectionBoxes_Precision/mAP (small): 0.6022
MASTERFUL [14:32:49]: DetectionBoxes_Precision/mAP (medium): 0.8318
MASTERFUL [14:32:49]: DetectionBoxes_Precision/mAP (large): 0.9343
MASTERFUL [14:32:49]: DetectionBoxes_Recall/AR@1: 0.6101
MASTERFUL [14:32:49]: DetectionBoxes_Recall/AR@10: 0.7949
MASTERFUL [14:32:49]: DetectionBoxes_Recall/AR@100: 0.7949
MASTERFUL [14:32:49]: DetectionBoxes_Recall/AR@100 (small): 0.6781
MASTERFUL [14:32:49]: DetectionBoxes_Recall/AR@100 (medium): 0.8705
MASTERFUL [14:32:49]: DetectionBoxes_Recall/AR@100 (large): 0.9479
MASTERFUL [14:32:49]: DetectionBoxes_PerformanceByCategory/mAP/0: 0.7744
MASTERFUL [14:32:49]: DetectionBoxes_PerformanceByCategory/mAP/1: 0.7481
MASTERFUL [14:32:49]: DetectionBoxes_PerformanceByCategory/mAP/2: 0.7269
MASTERFUL [14:32:49]: DetectionBoxes_PerformanceByCategory/mAP/3: 0.7851
MASTERFUL [14:32:49]: DetectionBoxes_PerformanceByCategory/mAP/4: 0.7444
MASTERFUL [14:32:49]: DetectionBoxes_PerformanceByCategory/mAP/5: 0.7149
MASTERFUL [14:32:49]: DetectionBoxes_PerformanceByCategory/mAP/6: 0.7831
MASTERFUL [14:32:49]: DetectionBoxes_PerformanceByCategory/mAP/7: 0.7767
MASTERFUL [14:32:49]: DetectionBoxes_PerformanceByCategory/mAP/8: 0.6802
MASTERFUL [14:32:49]: DetectionBoxes_PerformanceByCategory/mAP/9: 0.7204
The primary aggregated metric above is DetectionBoxes_Precision/mAP
, which is the Average Precision calculated over all of the IoU thresholds. Secondarily, the DetectionBoxes_Precision/mAP@.50IOU
corresponds to the Average Precision at just the 0.5 IoU threshold, which is a good intuitive indicator of performance and corresponds to the older Pascal VOC object detection metrics. The models scores relatively high on both of these metrics (0.7454
and 0.9290
) respectively. As you can
see in the per-category metrics (DetectionBoxes_PerformanceByCategory
), the model scores decently well across all of the different classes, with the worst performance on digit 8
and the best performance on digit 3
.
Confusion Matrix¶
The final set of metrics Masterful provides for Object Detection is the confusion matrix of predictions versus labels. The confusion matrix helps figure out where and how the model makes mistakes.
Below is the output for the confusion matrix from the training process:
MASTERFUL [14:33:00]: Confusion Matrix:
MASTERFUL [14:33:00]: | 0| 1| 2| 3| 4| 5| 6| 7| 8| 9| background|
MASTERFUL [14:33:00]: 0| 64| 0| 0| 0| 0| 0| 0| 0| 1| 0| 5|
MASTERFUL [14:33:00]: 1| 0| 55| 0| 0| 0| 0| 0| 0| 0| 0| 2|
MASTERFUL [14:33:00]: 2| 0| 0| 34| 5| 0| 0| 0| 0| 0| 1| 4|
MASTERFUL [14:33:00]: 3| 0| 0| 2| 57| 0| 0| 0| 0| 0| 1| 2|
MASTERFUL [14:33:00]: 4| 0| 1| 0| 0| 59| 0| 1| 2| 0| 5| 6|
MASTERFUL [14:33:00]: 5| 0| 0| 1| 0| 1| 53| 0| 0| 1| 0| 4|
MASTERFUL [14:33:00]: 6| 0| 1| 0| 0| 0| 0| 62| 0| 1| 0| 2|
MASTERFUL [14:33:00]: 7| 0| 1| 2| 1| 1| 0| 0| 63| 0| 6| 4|
MASTERFUL [14:33:00]: 8| 1| 0| 0| 2| 0| 2| 2| 0| 40| 3| 5|
MASTERFUL [14:33:00]: 9| 0| 0| 0| 1| 2| 0| 0| 1| 0| 49| 6|
MASTERFUL [14:33:00]: background| 0| 2| 2| 4| 3| 2| 0| 4| 2| 4| 0|
MASTERFUL [14:33:00]: Confusion matrix columns represent the prediction labels and the rows represent
the real labels.
In the above output, the columns represent the predictions made for the model. So the 3
column represents all of the examples where the model predicted 3
. For this column, each row represents the label in the dataset. So for the 3
column, the row 3
represents all of the examples where the model predicted 3
and the label in the dataset was also 3
. These are considered the true positives - the examples where the model made a correct positive prediction. In the 3
column, the 7
row represents the examples where the model predicted 3
, but the true label was actually 7
. These are considered false positives - examples where the model correctly detected an object but predicted the wrong class. However, because a detection task requires both correctly identifying the presence of an object as well as correctly classifying the type of that object, it is possible that the model predicts the existence of an object that does not exist. This is
quantified in the background
row of the above matrix. For example, In the 3
column, the background
cell represents all of the examples where the model predicted a 3
, but there was no actual 3
in that location or image. These are false postives where the model predicted the incorrect existence of a 3
, rather than correctly predicting the existence but mis-categorizing the object instance. But if the background
row contains predictions where there should not have
been any, what does the background
column represent? The background
column represents predictions that were not made. In other words, for the background
column and 3
row, this cell represents the examples where a 3
existed but the model failed to predict a 3
at this position. This represents a false negative
prediction from the model.
As you can see, the diagonal of the confusion matrix represents all of the correct positive predictions of the model. The off-diagonals represent different mistakes the model made in its predictions.
In the above explanation, you have see the location of the true positives
(the diagonals) and the location of the false positives
(off-diagonals) and false negatives
(background
column). One question you might ask, is where are the true negatives
- the examples where there were no instances of an object and the model correctly made no predictions? There are two ways to interpret false negatives
from the above matrix. The first is as true positives
on the
background
class - the diagonal entry in the background
column and row. These are the instances in the dataset with no objects and the model correctly predicted no objects. In this dataset however, every example has an object. You can see this in the test dataset analysis output for the Num Empty Images (No Labels)
row:
MASTERFUL [12:18:08]: Test dataset analysis
----------------------------- ----------------------------------------
Total Examples 100
Label Counts 0 70
1 57
2 44
3 62
4 74
5 60
6 66
7 78
8 55
9 59
.
.
.
Num Empty Images (No Labels): 0
Total Objects 625
Another way to categorize true negatives
is that every true positive
on one class is a corresponding true negative
on another class - correctly predicting the existence of one class is also correctly predicting the absence of another class, or all other classes. However, neither of these definitions provide much value, and importantly they can overestimate derived metrics. For example, accuracy
is commonly defined as the number of “correct” predictions (true positives
plus
true negatives
) over the total number of objects. In object detection however, this will drastically over-estimate the accuracy of the model given the presence of the true negatives
term, which when averaged over all of the classes you would most likely end up with more true negatives
than object instances in the dataset. This is historically why object detection has relied on precision
and recall
metrics, rather than accuracy
, due to the lack of a true negative
term
in the definition of the former.
View the Predictions¶
While the mAP metrics are a good quantitative indicator of the performance of your model, visualizing the predictions can help you get a qualititative sense of how well your trained model is performing. Below you can see the predictions for the above trained model has on the examples you visualized above. The red boxes are the labels from the dataset, and the green boxes are the predictions made by the model.
[5]:
# Download the pretrained model so that you can
# visualize the results.
MODEL_URL = "https://masterful-public.s3.us-west-1.amazonaws.com/datasets/yymnist/yymnist_no_unlabeled_saved_model.tar.gz"
model = None
with tempfile.TemporaryDirectory() as temp_directory:
# Download the pretrained model from S3.
saved_model_path = os.path.join(temp_directory, "saved_model.tar.gz")
_ = urllib.request.urlretrieve(MODEL_URL, saved_model_path, progress_bar_factory("Downloading Model: "))
# Extract the model weights from the tar file.
with tarfile.open(saved_model_path) as tar:
tar.extractall(temp_directory)
saved_model_path = os.path.join(temp_directory, "saved_model")
# Load the trained tensorflow saved model.
model = tf.saved_model.load(saved_model_path)
# Plot a few different instances from the dataset.
f, axarr = plt.subplots(2, 2, figsize=(15,15))
_ = axarr[0, 0].imshow(create_annotated_image(examples[0], DATASET_ROOT, model))
_ = axarr[0, 1].imshow(create_annotated_image(examples[1], DATASET_ROOT, model))
_ = axarr[1, 0].imshow(create_annotated_image(examples[2], DATASET_ROOT, model))
_ = axarr[1, 1].imshow(create_annotated_image(examples[3], DATASET_ROOT, model))
Downloading Model: 100% (19166150 of 19166150) || Elapsed Time: 0:00:01 Time: 0:00:01
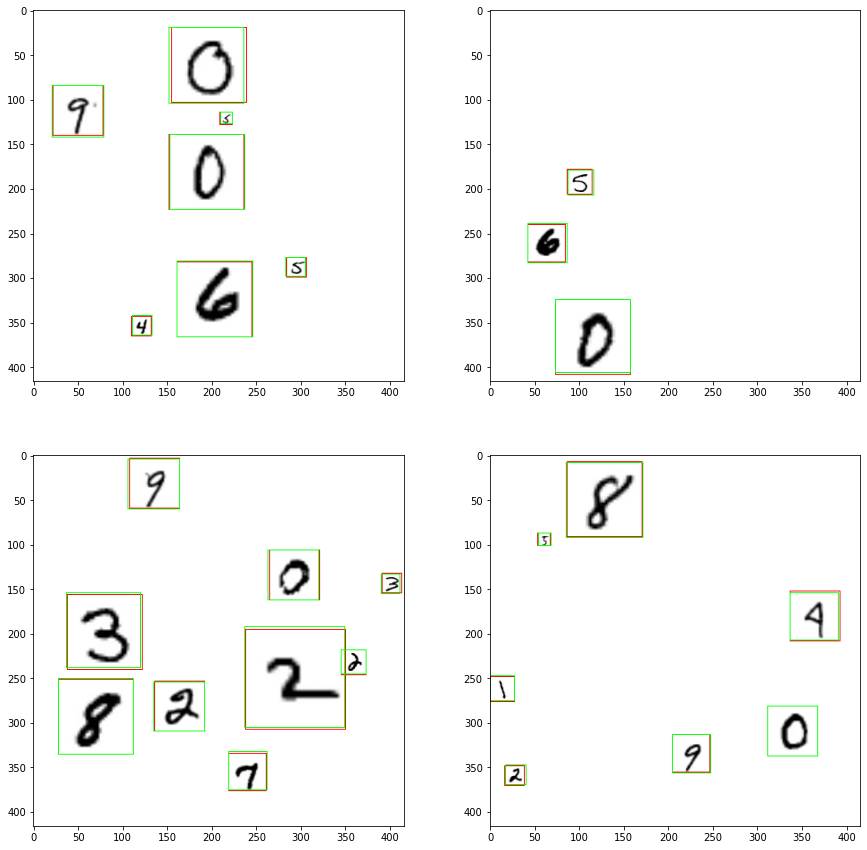
As you can see in the above predictions, the model performs exceptionally well in localizing all of the the different digits! The predicted bounding boxes (green) are tight against the ground truth bounding boxes (red) and no digits are missing and there are no predictions in areas with no digits.
Improve Your Model with Unlabeled Data¶
The performance of your model above was great, but can you do better? The answer is yes, by adding unlabeled data! Below, you will retrain your model with an additional unlabeled data split. Adding unlabeled data is easy. The CSV file just lists the images without any labels. For example, here are the first few items from unlabeled.csv, which lists all of the unlabeled training items.
images/extra/001001.jpg
images/extra/001002.jpg
images/extra/001003.jpg
images/extra/001004.jpg
images/extra/001005.jpg
images/extra/001006.jpg
images/extra/001007.jpg
images/extra/001008.jpg
images/extra/001009.jpg
images/extra/001010.jpg
.
.
.
You will also point to a new YAML file training_with_unlabeled.yaml which add the above unlabeled data to the training process. There are only two differences in this yaml versus the one you just trained with. First, you let the dataset configuration know about the unlabeled data:
dataset:
splits: [train, test, unlabeled]
Second, you tell the training process to use that split:
training:
task: detection
training_split: train
unlabeled_split: unlabeled
If you want to run the trainer with unlabeled data outside of this notebook, you can simply run the following command:
masterful-train --config https://masterful-public.s3.us-west-1.amazonaws.com/datasets/yymnist/training_with_unlabeled.yaml
Otherwise, you can run the following cell:
[2]:
# Use the Masterful CLI to train the model WITH unlabeled data
!masterful-train --config https://masterful-public.s3.us-west-1.amazonaws.com/datasets/yymnist/training_with_unlabeled.yaml
MASTERFUL: Your account has been successfully activated. Masterful v0.5.2 is loaded.
MASTERFUL [14:34:42]: Training with configuration 'https://masterful-public.s3.us-west-1.amazonaws.com/datasets/yymnist/training_with_unlabeled.yaml':
---------- ----------------------------------------------------------------------------------
dataset root_path https://masterful-public.s3.us-west-1.amazonaws.com/datasets/yymnist
splits ['train', 'test', 'unlabeled']
label_format detection_csv
optimize True
model architecture ssd_mobilenet_v2
num_classes 10
input_shape [416, 416, 3]
training task detection
training_split train
unlabeled_split unlabeled
output formats ['saved_model', 'onnx']
path ~/model_output
evaluation split test
---------- ----------------------------------------------------------------------------------
MASTERFUL [14:34:43]: Building model 'ssd_mobilenet_v2'...
MASTERFUL [14:34:47]: Using model ssd_mobilenet_v2 with:
MASTERFUL [14:34:47]: 4795759 total parameters
MASTERFUL [14:34:47]: 4758191 trainable parameters
MASTERFUL [14:34:47]: 37568 untrainable parameters
MASTERFUL [14:34:50]: Dataset Summary:
MASTERFUL [14:34:50]: Training Dataset: 900 examples.
MASTERFUL [14:34:50]: Validation Dataset: 0 examples.
MASTERFUL [14:34:50]: Test Dataset: 100 examples.
MASTERFUL [14:34:50]: Unlabeled Dataset: 9000 examples.
MASTERFUL [14:34:50]: Training Dataset Analysis:
100%|█████████████████████████████████████████| 900/900 [00:16<00:00, 53.96it/s]
MASTERFUL [14:35:07]: Training dataset analysis finished at 14:35:07 in 17 seconds (17s), returned:
----------------------------- ----------------------------------------
Total Examples 900
Label Counts 0 494
1 616
2 552
3 533
4 522
5 440
6 581
7 555
8 491
9 549
Label Distribution 0 0.0926308
1 0.115507
2 0.103506
3 0.0999437
4 0.0978811
5 0.0825052
6 0.108944
7 0.104069
8 0.0920683
9 0.102944
Balanced Yes
Per Channel Mean [251.53575897 251.53575897 251.53575897]
Per Channel StdDev [26.2797294 26.2797294 26.2797294]
Min Height 416
Min Width 416
Max Height 416
Max Width 416
Average Height 416
Average Width 416
Largest Image (416, 416, 3)
Smallest Image (416, 416, 3)
Duplicates 0
Average Object Area 3565
Smallest Object Area 195
Largest Object Area 12544
Num Small Objects 2216
Num Medium Objects 2415
Num Large Objects 702
Num Empty Images (No Labels): 0
Total Objects 5333
----------------------------- ----------------------------------------
MASTERFUL [14:35:07]: Test Dataset Analysis:
100%|█████████████████████████████████████████| 100/100 [00:01<00:00, 50.68it/s]
MASTERFUL [14:35:09]: Test dataset analysis finished at 14:35:09 in 2 seconds (2s), returned:
----------------------------- ----------------------------------------
Total Examples 100
Label Counts 0 70
1 57
2 44
3 62
4 74
5 60
6 66
7 78
8 55
9 59
Label Distribution 0 0.112
1 0.0912
2 0.0704
3 0.0992
4 0.1184
5 0.096
6 0.1056
7 0.1248
8 0.088
9 0.0944
Balanced Yes
Per Channel Mean [251.62994095 251.62994095 251.62994095]
Per Channel StdDev [26.10288871 26.10288871 26.10288871]
Min Height 416
Min Width 416
Max Height 416
Max Width 416
Average Height 416
Average Width 416
Largest Image (416, 416, 3)
Smallest Image (416, 416, 3)
Duplicates 0
Average Object Area 3259
Smallest Object Area 195
Largest Object Area 12544
Num Small Objects 270
Num Medium Objects 290
Num Large Objects 65
Num Empty Images (No Labels): 0
Total Objects 625
----------------------------- ----------------------------------------
MASTERFUL [14:35:09]: Unlabeled Dataset Analysis:
100%|███████████████████████████████████████| 9000/9000 [01:30<00:00, 99.26it/s]
MASTERFUL [14:36:39]: Unlabeled dataset analysis finished at 14:36:39 in 91 seconds (1m 31s), returned:
------------------ ----------------------------------------
Total Examples 9000
Per Channel Mean [251.44937014 251.44937014 251.44937014]
Per Channel StdDev [26.67446678 26.67446678 26.67446678]
Min Height 416
Min Width 416
Max Height 416
Max Width 416
Average Height 416
Average Width 416
Largest Image (416, 416, 3)
Smallest Image (416, 416, 3)
Duplicates 0
------------------ ----------------------------------------
MASTERFUL [14:36:39]: Cross-Dataset Analysis:
MASTERFUL [14:36:40]: Cross-Dataset analysis finished at 14:36:40 in 0 seconds (0s), returned:
--------- ------------
train train 0
test 0
unlabeled 0
test train 0
test 0
unlabeled 0
unlabeled train 0
test 0
unlabeled 0
--------- ------------
MASTERFUL [14:36:40]: Meta-Learning architecture parameters...
MASTERFUL [14:36:40]: Architecture learner finished at 14:36:40 in 0 seconds (0s), returned:
------------------------------ -----------------------------------------------------
task Task.DETECTION
num_classes 10
ensemble_multiplier 1
custom_objects
model_config ssd {
num_classes: 10
image_resizer {
fixed_shape_resizer {
height: 416
width: 416
}
}
feature_extractor {
type: "ssd_mobilenet_v2_keras"
depth_multiplier: 1.0
min_depth: 16
conv_hyperparams {
regularizer {
l2_regularizer {
weight: 3.9999998989515007e-05
}
}
initializer {
truncated_normal_initializer {
mean: 0.0
stddev: 0.029999999329447746
}
}
activation: RELU_6
batch_norm {
decay: 0.9700000286102295
center: true
scale: true
epsilon: 0.0010000000474974513
train: true
}
}
override_base_feature_extractor_hyperparams: true
}
box_coder {
faster_rcnn_box_coder {
y_scale: 10.0
x_scale: 10.0
height_scale: 5.0
width_scale: 5.0
}
}
matcher {
argmax_matcher {
matched_threshold: 0.5
unmatched_threshold: 0.5
ignore_thresholds: false
negatives_lower_than_unmatched: true
force_match_for_each_row: true
use_matmul_gather: true
}
}
similarity_calculator {
iou_similarity {
}
}
box_predictor {
convolutional_box_predictor {
conv_hyperparams {
regularizer {
l2_regularizer {
weight: 3.9999998989515007e-05
}
}
initializer {
random_normal_initializer {
mean: 0.0
stddev: 0.009999999776482582
}
}
activation: RELU_6
batch_norm {
decay: 0.9700000286102295
center: true
scale: true
epsilon: 0.0010000000474974513
train: true
}
}
min_depth: 0
max_depth: 0
num_layers_before_predictor: 0
use_dropout: false
dropout_keep_probability: 0.800000011920929
kernel_size: 1
box_code_size: 4
apply_sigmoid_to_scores: false
class_prediction_bias_init: -4.599999904632568
}
}
anchor_generator {
ssd_anchor_generator {
num_layers: 6
min_scale: 0.20000000298023224
max_scale: 0.949999988079071
aspect_ratios: 1.0
aspect_ratios: 2.0
aspect_ratios: 0.5
aspect_ratios: 3.0
aspect_ratios: 0.33329999446868896
}
}
post_processing {
batch_non_max_suppression {
score_threshold: 9.99999993922529e-09
iou_threshold: 0.6000000238418579
max_detections_per_class: 100
max_total_detections: 100
}
score_converter: SIGMOID
}
normalize_loss_by_num_matches: true
loss {
localization_loss {
weighted_smooth_l1 {
delta: 1.0
}
}
classification_loss {
weighted_sigmoid_focal {
gamma: 2.0
alpha: 0.75
}
}
classification_weight: 1.0
localization_weight: 1.0
}
encode_background_as_zeros: true
normalize_loc_loss_by_codesize: true
inplace_batchnorm_update: true
freeze_batchnorm: false
}
backbone_only False
input_shape (416, 416, 3)
input_range ImageRange.ZERO_255
input_dtype <dtype: 'float32'>
input_channels_last True
prediction_logits True
prediction_dtype <dtype: 'float32'>
prediction_structure TensorStructure.DICT
prediction_shape
total_parameters
total_trainable_parameters
total_non_trainable_parameters
------------------------------ -----------------------------------------------------
MASTERFUL [14:36:40]: Meta-learning training dataset parameters...
MASTERFUL [14:36:41]: Training dataset learner finished at 14:36:41 in 1 seconds (1s), returned:
------------------------- -----------------------------
num_classes 10
task Task.DETECTION
image_shape (416, 416, 3)
image_range ImageRange.ZERO_255
image_dtype <dtype: 'float32'>
image_channels_last True
label_dtype <dtype: 'float32'>
label_shape (12, 15)
label_structure TensorStructure.SINGLE_TENSOR
label_sparse False
label_bounding_box_format BoundingBoxFormat.TENSORFLOW
------------------------- -----------------------------
MASTERFUL [14:36:41]: Meta-learning test dataset parameters...
MASTERFUL [14:36:41]: Test dataset learner finished at 14:36:41 in 1 seconds (1s), returned:
------------------------- -----------------------------
num_classes 10
task Task.DETECTION
image_shape (416, 416, 3)
image_range ImageRange.ZERO_255
image_dtype <dtype: 'float32'>
image_channels_last True
label_dtype <dtype: 'float32'>
label_shape (11, 15)
label_structure TensorStructure.SINGLE_TENSOR
label_sparse False
label_bounding_box_format BoundingBoxFormat.TENSORFLOW
------------------------- -----------------------------
MASTERFUL [14:36:41]: Meta-learning unlabeled dataset parameters...
MASTERFUL [14:36:42]: Unlabeled dataset learner finished at 14:36:42 in 1 seconds (1s), returned:
------------------------- -------------------
num_classes 10
task Task.DETECTION
image_shape (416, 416, 3)
image_range ImageRange.ZERO_255
image_dtype <dtype: 'float32'>
image_channels_last True
label_dtype
label_shape
label_structure
label_sparse False
label_bounding_box_format
------------------------- -------------------
MASTERFUL [14:36:42]: Meta-Learning optimization parameters...
Callbacks: 100%|███████████████████████████████| 5/5 [01:25<00:00, 17.13s/steps]
MASTERFUL [14:38:08]: Optimization learner finished at 14:38:08 in 86 seconds (1m 26s), returned:
----------------------- -----------------------------------------------------------------
batch_size 16
drop_remainder True
epochs 1000000
learning_rate 0.0008838834473863244
learning_rate_schedule
learning_rate_callback <keras.callbacks.ReduceLROnPlateau object at 0x7fad1505def0>
warmup_learning_rate 1e-06
warmup_epochs 5
optimizer <tensorflow_addons.optimizers.lamb.LAMB object at 0x7facfc0d0c50>
loss
loss_weights
early_stopping_callback <keras.callbacks.EarlyStopping object at 0x7facfc0d0d30>
metrics
readonly_callbacks
----------------------- -----------------------------------------------------------------
MASTERFUL [14:38:10]: Meta-Learning Regularization Parameters...
MASTERFUL [14:38:24]: Warming up model for analysis.
MASTERFUL [14:38:25]: Warming up batch norm statistics (this could take a few minutes).
MASTERFUL [14:38:28]: Warming up training for 250 steps.
100%|██████████████████████████████████████| 250/250 [01:56<00:00, 2.15steps/s]
MASTERFUL [14:40:24]: Validating batch norm statistics after warmup for stability (this could take a few minutes).
MASTERFUL [14:40:25]: Analyzing baseline model performance. Training until validation loss stabilizes...
Baseline Training: 100%|█████████████████| 2365/2365 [09:40<00:00, 4.08steps/s]
MASTERFUL [14:50:27]: Baseline training complete.
MASTERFUL [14:50:28]: Meta-Learning Basic Data Augmentations...
Node 1/4: 100%|██████████████████████████| 1100/1100 [04:28<00:00, 4.09steps/s]
Node 2/4: 100%|██████████████████████████| 1100/1100 [04:36<00:00, 3.98steps/s]
Node 3/4: 100%|██████████████████████████| 1100/1100 [04:29<00:00, 4.08steps/s]
Node 4/4: 100%|██████████████████████████| 1100/1100 [04:29<00:00, 4.08steps/s]
MASTERFUL [15:09:52]: Meta-Learning Data Augmentation Clusters...
Distance Analysis: 100%|███████████████████| 143/143 [02:19<00:00, 1.02steps/s]
Node 1/10: 100%|█████████████████████████| 1100/1100 [05:58<00:00, 3.06steps/s]
Node 2/10: 100%|█████████████████████████| 1100/1100 [05:59<00:00, 3.06steps/s]
Node 3/10: 100%|█████████████████████████| 1100/1100 [05:59<00:00, 3.06steps/s]
Node 4/10: 100%|█████████████████████████| 1100/1100 [05:59<00:00, 3.06steps/s]
Node 5/10: 100%|█████████████████████████| 1100/1100 [05:59<00:00, 3.06steps/s]
Distance Analysis: 100%|█████████████████████| 66/66 [01:06<00:00, 1.00s/steps]
Node 6/10: 100%|█████████████████████████| 1100/1100 [05:02<00:00, 3.63steps/s]
Node 7/10: 100%|█████████████████████████| 1100/1100 [05:14<00:00, 3.50steps/s]
Node 8/10: 100%|█████████████████████████| 1100/1100 [05:12<00:00, 3.52steps/s]
Node 9/10: 100%|█████████████████████████| 1100/1100 [05:01<00:00, 3.65steps/s]
Node 10/10: 100%|████████████████████████| 1100/1100 [04:51<00:00, 3.77steps/s]
MASTERFUL [16:12:02]: Meta-Learning Label Based Regularization...
Node 1/2: 100%|██████████████████████████| 1100/1100 [04:32<00:00, 4.04steps/s]
Node 2/2: 100%|██████████████████████████| 1100/1100 [04:34<00:00, 4.01steps/s]
MASTERFUL [16:21:42]: Meta-Learning Weight Based Regularization...
MASTERFUL [16:21:43]: Analysis finished in 103.36468438307445 minutes.
MASTERFUL [16:21:43]: Learned parameters scene-delicious-gander saved at /home/sam/.masterful/policies/scene-delicious-gander.
MASTERFUL [16:21:43]: Regularization learner finished at 16:21:43 in 6214 seconds (1h 43m 34s), returned:
------------------------- -----------------------------------------------
shuffle_buffer_size 810
mirror 1.0
rot90 0.0
rotate 0
mixup 0.75
cutmix 0.0
label_smoothing 0
hsv_cluster 0
hsv_cluster_to_index [[11 11 11 11 11 11]
[11 11 11 11 11 11]
[11 11 11 11 11 11]
[11 11 11 11 11 11]
[11 11 11 11 11 11]]
hsv_magnitude_table [[ 0 10 20 30 40 50 60 70 80 90 100]
[ 0 10 20 30 40 50 60 70 80 90 100]
[ 0 10 20 30 40 50 60 70 80 90 100]
[ 0 10 20 30 40 50 60 70 80 90 100]
[ 0 10 20 30 40 50 60 70 80 90 100]]
contrast_cluster 0
contrast_cluster_to_index [[11 11 11 11 11 11]
[11 11 11 11 11 11]
[11 11 11 11 11 11]
[11 11 11 11 11 11]
[11 11 11 11 11 11]
[11 11 11 11 11 11]]
contrast_magnitude_table [[ 0 10 20 30 40 50 60 70 80 90 100]
[ 0 10 20 30 40 50 60 70 80 90 100]
[ 0 10 20 30 40 50 60 70 80 90 100]
[ 0 10 20 30 40 50 60 70 80 90 100]
[ 0 10 20 30 40 50 60 70 80 90 100]
[ 0 10 20 30 40 50 60 70 80 90 100]]
blur_cluster 0
blur_cluster_to_index [[11 11 11 11 11 11]
[11 11 11 11 11 11]]
blur_magnitude_table [[ 0 10 20 30 40 50 60 70 80 90 100]
[ 0 10 20 30 40 50 60 70 80 90 100]]
spatial_cluster 0
spatial_cluster_to_index [[11 11 11 11 11 11]
[11 11 11 11 11 11]
[11 11 11 11 11 11]
[11 11 11 11 11 11]
[11 11 11 11 11 11]
[11 11 11 11 11 11]]
spatial_magnitude_table [[ 0 10 20 30 40 50 60 70 80 90 100]
[ 0 10 20 30 40 50 60 70 80 90 100]
[ 0 10 20 30 40 50 60 70 80 90 100]
[ 0 10 20 30 40 50 60 70 80 90 100]
[ 0 10 20 30 40 50 60 70 80 90 100]
[ 0 10 20 30 40 50 60 70 80 90 100]]
synthetic_proportion [0.0]
------------------------- -----------------------------------------------
MASTERFUL [16:21:43]: Learning SSL parameters...
MASTERFUL [16:21:44]: SSL learner finished at 16:21:44 in 1 seconds (1s), returned:
------------------------- -------------------
num_classes 10
task Task.DETECTION
image_shape (416, 416, 3)
image_range ImageRange.ZERO_255
image_dtype <dtype: 'float32'>
image_channels_last True
label_dtype
label_shape
label_structure
label_sparse False
label_bounding_box_format
------------------------- -------------------
MASTERFUL [16:21:45]: Training model with semi-supervised learning enabled.
MASTERFUL [16:21:45]: Performing basic dataset analysis.
MASTERFUL [16:21:55]: Masterful will use 90 labeled examples as a validation set since no validation data was provided.
MASTERFUL [16:21:55]: Training model with:
MASTERFUL [16:21:55]: 810 labeled examples.
MASTERFUL [16:21:55]: 90 validation examples.
MASTERFUL [16:21:55]: 0 synthetic examples.
MASTERFUL [16:21:55]: 9000 unlabeled examples.
MASTERFUL [16:21:56]: Training model with learned parameters scene-delicious-gander in two phases.
MASTERFUL [16:21:56]: The first phase is supervised training with the learned parameters.
MASTERFUL [16:21:56]: The second phase is semi-supervised training to boost performance.
MASTERFUL [16:22:06]: Warming up model for supervised training.
MASTERFUL [16:22:08]: Warming up batch norm statistics (this could take a few minutes).
MASTERFUL [16:22:12]: Warming up training for 250 steps.
100%|██████████████████████████████████████| 250/250 [02:15<00:00, 1.84steps/s]
MASTERFUL [16:24:27]: Validating batch norm statistics after warmup for stability (this could take a few minutes).
MASTERFUL [16:24:29]: Starting Phase 1: Supervised training until the validation loss stabilizes...
Supervised Training: 100%|███████████████| 3300/3300 [22:20<00:00, 2.46steps/s]
MASTERFUL [16:47:18]: Starting Phase 2: Semi-supervised training until the validation loss stabilizes...
MASTERFUL [16:47:18]: Warming up model for semi-supervised training.
MASTERFUL [16:47:18]: Warming up batch norm statistics (this could take a few minutes).
MASTERFUL [16:47:19]: Warming up training for 250 steps.
100%|██████████████████████████████████████| 250/250 [01:48<00:00, 2.30steps/s]
MASTERFUL [16:49:08]: Validating batch norm statistics after warmup for stability (this could take a few minutes).
Semi-Supervised Training: 100%|██████| 16042/16042 [1:46:53<00:00, 2.50steps/s]
MASTERFUL [18:40:25]: Semi-Supervised training complete.
MASTERFUL [18:40:27]: Training complete in 138.50163372357687 minutes.
MASTERFUL [18:40:32]: Saving model output to /home/sam/model_output/session-00342.
MASTERFUL [18:40:42]: Saving saved_model output to /home/sam/model_output/session-00342/saved_model
MASTERFUL [18:41:18]: Saving onnx output to /home/sam/model_output/session-00342/onnx
MASTERFUL [18:42:23]: Saving regularization params to /home/sam/model_output/session-00342/regularization.params.
MASTERFUL [18:42:23]: ************************************
MASTERFUL [18:42:23]: Evaluating model on 100 examples from the 'test' dataset split:
Evaluating: 100%|█████████████████████████████| 100/100 [00:06<00:00, 16.39it/s]
MASTERFUL [18:42:29]: Loss: 0.1950
MASTERFUL [18:42:29]: Loss/Localization Loss: 0.0204
MASTERFUL [18:42:29]: Loss/Classification Loss: 0.1100
MASTERFUL [18:42:29]: Loss/Regularization Loss: 0.0647
MASTERFUL [18:42:29]: Loss/Total Loss: 0.1950
MASTERFUL [18:42:29]: Calculating MSCOCO evaluation metrics:
MSCOCO Metrics: 100%|█████████████████████████| 100/100 [00:14<00:00, 6.93it/s]
MASTERFUL [18:42:44]: DetectionBoxes_Precision/mAP: 0.7625
MASTERFUL [18:42:44]: DetectionBoxes_Precision/mAP@.50IOU: 0.9311
MASTERFUL [18:42:44]: DetectionBoxes_Precision/mAP@.75IOU: 0.8991
MASTERFUL [18:42:44]: DetectionBoxes_Precision/mAP (small): 0.6476
MASTERFUL [18:42:44]: DetectionBoxes_Precision/mAP (medium): 0.8352
MASTERFUL [18:42:44]: DetectionBoxes_Precision/mAP (large): 0.8990
MASTERFUL [18:42:44]: DetectionBoxes_Recall/AR@1: 0.6156
MASTERFUL [18:42:44]: DetectionBoxes_Recall/AR@10: 0.8163
MASTERFUL [18:42:44]: DetectionBoxes_Recall/AR@100: 0.8163
MASTERFUL [18:42:44]: DetectionBoxes_Recall/AR@100 (small): 0.7278
MASTERFUL [18:42:44]: DetectionBoxes_Recall/AR@100 (medium): 0.8780
MASTERFUL [18:42:44]: DetectionBoxes_Recall/AR@100 (large): 0.9138
MASTERFUL [18:42:44]: DetectionBoxes_PerformanceByCategory/mAP/0: 0.7844
MASTERFUL [18:42:44]: DetectionBoxes_PerformanceByCategory/mAP/1: 0.8332
MASTERFUL [18:42:44]: DetectionBoxes_PerformanceByCategory/mAP/2: 0.7457
MASTERFUL [18:42:44]: DetectionBoxes_PerformanceByCategory/mAP/3: 0.7791
MASTERFUL [18:42:44]: DetectionBoxes_PerformanceByCategory/mAP/4: 0.7758
MASTERFUL [18:42:44]: DetectionBoxes_PerformanceByCategory/mAP/5: 0.7530
MASTERFUL [18:42:44]: DetectionBoxes_PerformanceByCategory/mAP/6: 0.7673
MASTERFUL [18:42:44]: DetectionBoxes_PerformanceByCategory/mAP/7: 0.7604
MASTERFUL [18:42:44]: DetectionBoxes_PerformanceByCategory/mAP/8: 0.7213
MASTERFUL [18:42:44]: DetectionBoxes_PerformanceByCategory/mAP/9: 0.7052
Confusion Matrix: 100%|███████████████████████| 100/100 [00:09<00:00, 10.13it/s]
MASTERFUL [18:42:54]: Confusion Matrix:
MASTERFUL [18:42:54]: | 0| 1| 2| 3| 4| 5| 6| 7| 8| 9| background|
MASTERFUL [18:42:54]: 0| 65| 0| 0| 0| 0| 0| 1| 0| 2| 1| 1|
MASTERFUL [18:42:54]: 1| 0| 56| 0| 0| 0| 0| 0| 0| 0| 0| 1|
MASTERFUL [18:42:54]: 2| 0| 0| 37| 3| 0| 0| 0| 0| 3| 0| 1|
MASTERFUL [18:42:54]: 3| 0| 0| 0| 58| 0| 1| 0| 0| 0| 0| 3|
MASTERFUL [18:42:54]: 4| 0| 0| 1| 0| 63| 0| 0| 1| 1| 4| 4|
MASTERFUL [18:42:54]: 5| 0| 0| 0| 3| 0| 56| 0| 0| 0| 0| 1|
MASTERFUL [18:42:54]: 6| 5| 0| 0| 0| 0| 0| 54| 0| 5| 0| 2|
MASTERFUL [18:42:54]: 7| 0| 0| 0| 1| 1| 0| 0| 63| 2| 10| 1|
MASTERFUL [18:42:54]: 8| 3| 0| 1| 2| 0| 1| 1| 0| 44| 1| 2|
MASTERFUL [18:42:54]: 9| 1| 0| 0| 1| 0| 1| 0| 2| 1| 49| 4|
MASTERFUL [18:42:54]: background| 5| 2| 4| 5| 3| 5| 2| 7| 9| 15| 0|
MASTERFUL [18:42:54]: Confusion matrix columns represent the prediction labels and the rows represent the real labels.
MASTERFUL [18:42:54]: Saving evaluation metrics to /home/sam/model_output/session-00342/evaluation_metrics.csv.
MASTERFUL [18:42:54]: Saving confusion matrix to /home/sam/model_output/session-00342/confusion_matrix.csv.
MASTERFUL [18:42:54]: Total elapsed training time: 248 minutes (4h 8m 11s).
MASTERFUL [18:42:54]: Launch masterful-gui to visualize the training results: policy name 'scene-delicious-gander'
Unlabeled Data Improved Model Training¶
In the below table is a summary of the evaluation metrics from both training runs, with and without unlabeled data:
Metric |
Without Unlabeled Data |
With Unlabeled Data |
---|---|---|
|
0.2189 |
0.1950 |
|
0.7454 |
0.7625 |
|
0.9290 |
0.9311 |
|
0.7744 |
0.7844 |
|
0.7481 |
0.8332 |
|
0.7269 |
0.7457 |
|
0.7851 |
0.7791 |
|
0.7444 |
0.7758 |
|
0.7149 |
0.7530 |
|
0.7831 |
0.7673 |
|
0.7767 |
0.7604 |
|
0.6802 |
0.7213 |
|
0.7204 |
0.7052 |
As you can see, the overall loss improved and the mAP metrics improved. From the per-category metrics, the model significantly improved on the worst performing classes. It did this at a small expense to the best-performing classes, which is okay because the model was already good at those, and by improving the worst performing classes the model got better overall!
Using the Model for Inference¶
The Output Formats guide has more information about how to use the models output by Masterful. Object Detection has a few other intricacies that should be addressed though, since the model outputs both the location of an object, a class instance for that object, and a measure of the confidence in the prediction. First, the below code shows how to make a prediction using the model we loaded above on the examples you visualized in the View the Predictions section:
[9]:
# This image corresponds to the first image (upper left)
# in the `View the Predictions` section above.
IMAGE_URL = "https://masterful-public.s3.us-west-1.amazonaws.com/datasets/yymnist/images/train/000001.jpg"
# Load the trained model so that you can test running inference.
MODEL_URL = "https://masterful-public.s3.us-west-1.amazonaws.com/datasets/yymnist/yymnist_with_unlabeled_saved_model.tar.gz"
model = None
with tempfile.TemporaryDirectory() as temp_directory:
# Download the pretrained model from S3.
saved_model_path = os.path.join(temp_directory, "saved_model.tar.gz")
_ = urllib.request.urlretrieve(MODEL_URL, saved_model_path, progress_bar_factory("Downloading Model: "))
# Extract the model weights from the tar file.
with tarfile.open(saved_model_path) as tar:
tar.extractall(temp_directory)
saved_model_path = os.path.join(temp_directory, "saved_model")
# Load the trained tensorflow saved model.
model = tf.saved_model.load(saved_model_path)
# Download the image to use for generating a prediction
# and convert it into a tensor.
http_response = requests.get(IMAGE_URL, stream=True)
image = tf.io.decode_image(http_response.raw.read())
image_height = image.shape[0]
image_width = image.shape[1]
print(f"Image Shape: height={image_height} width={image_width}.")
# The default inference function for tensorflow saved
# models is named `serving_default`.
inference_fn = model.signatures["serving_default"]
# The inference function is a one-arg callable, whose
# input argument is `image` - the image to predict on,
# and which returns a dictionary of outputs. The dictionary
# contains an item whose key is `prediction`, which is the
# predictions of the model.
predictions = inference_fn(image=image)['prediction']
# Convert the predictions to NumPY for easier processing.
predictions = predictions.numpy()
# The predictions from an object detection model
# have the shape [number of boxes, 6].
# The number of boxes predicted depends on the model
# configuration and represents the maximum number of boxes
# predicted for each example. A typical value is 100.
print(f"Predictions shape is {predictions.shape}")
print(f"Maximum number of boxes predicted is {predictions.shape[0]}")
# The final dimension of the prediction represents the boxes,
# classes, and confidences predicted by the model. This dimension
# is of form: [ymin, xmin, ymax, xmax, class id, confidence].
# The box values are normalized against the image size, and are
# in the range [0,1].
#
# The confidence score is a value in the range [0,1] which represents
# the confidence of the prediction, similar to the confidence
# of a classification model represented by the softmax probabities
# of the class predictions. The step to determingin the `true`
# predictions is to threshold the predictions by the confidence
# score. A typical scheme is to use only the predictions whose
# confidence is greater than 0.5 as the threshold, but you can
# experiment with different threshold values for your use case.
threshold = 0.5
true_predictions = predictions[predictions[:, 5] > threshold]
print(f"Found {true_predictions.shape[0]} confident predictions out of {predictions.shape[0]} total predictions.")
# Print the model predictions above the given threshold
for i in range(true_predictions.shape[0]):
print(f"Prediction {i}:")
print(f" Class: {int(true_predictions[i][4])}")
print(f" Left: {int(true_predictions[i][1]*image_width)}")
print(f" Top: {int(true_predictions[i][0]*image_height)}")
print(f" Right: {int(true_predictions[i][3]*image_width)}")
print(f" Bottom: {int(true_predictions[i][2]*image_height)}")
Downloading Model: 100% (19376673 of 19376673) || Elapsed Time: 0:00:02 Time: 0:00:02
Image Shape: height=416 width=416.
Predictions shape is (1, 100, 6)
Maximum number of boxes predicted is 100
Found 6 confident predictions out of 100 total predictions.
Prediction 0:
Class: 0
Left: 154
Top: 137
Right: 241
Bottom: 223
Prediction 1:
Class: 6
Left: 162
Top: 282
Right: 247
Bottom: 366
Prediction 2:
Class: 0
Left: 155
Top: 21
Right: 241
Bottom: 106
Prediction 3:
Class: 5
Left: 284
Top: 277
Right: 306
Bottom: 299
Prediction 4:
Class: 5
Left: 208
Top: 115
Right: 223
Bottom: 130
Prediction 5:
Class: 9
Left: 23
Top: 84
Right: 78
Bottom: 140
Prediction 6:
Class: 4
Left: 109
Top: 342
Right: 132
Bottom: 365
Next Steps¶
In the above sections, you have learned how to train an object detection model using the Masterful CLI, evaluate the performance of the model, and use the model to make predictions on your production data.
If you want to look at another, more involved Object Detection example, see Detecting Pedestrians in Street Level Imagery for more information. Otherwise, feel free to explore Classifying Land Use and Segmenting Animals for other examples of using the Masterful CLI Trainer to solve challenging computer vision problems.